Biostatistics :
Statistics Applied to Biological Sciences
Around the 20th Century, biologists faced growing data complexities. This led to the emergence of biostatistics, applying statistical methods to biological sciences.
While biostatistics overlaps with statistics, it often involves methods tailored to biological inquiries.
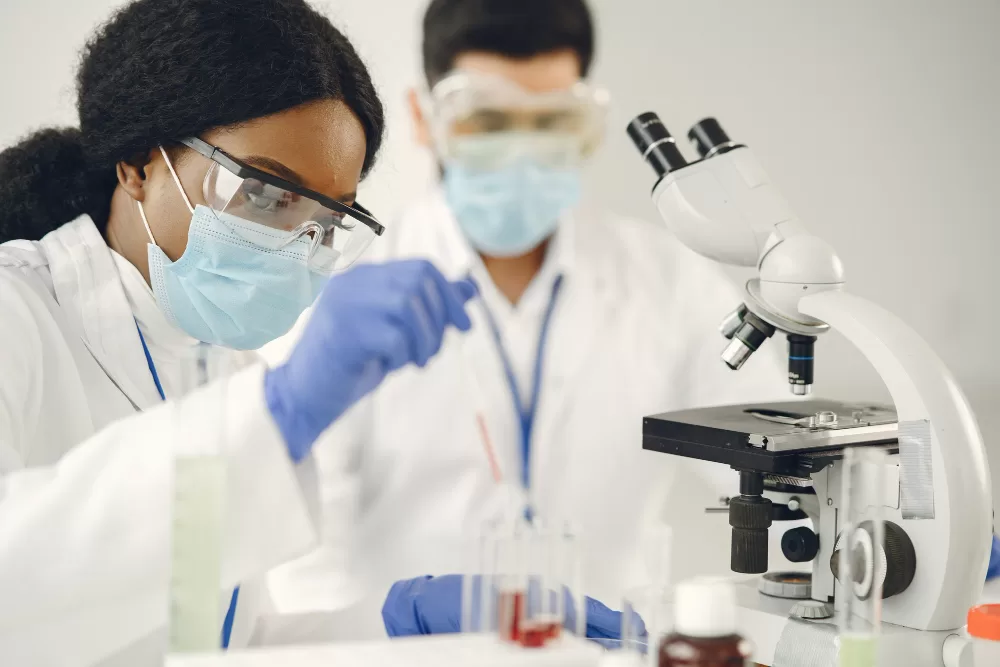
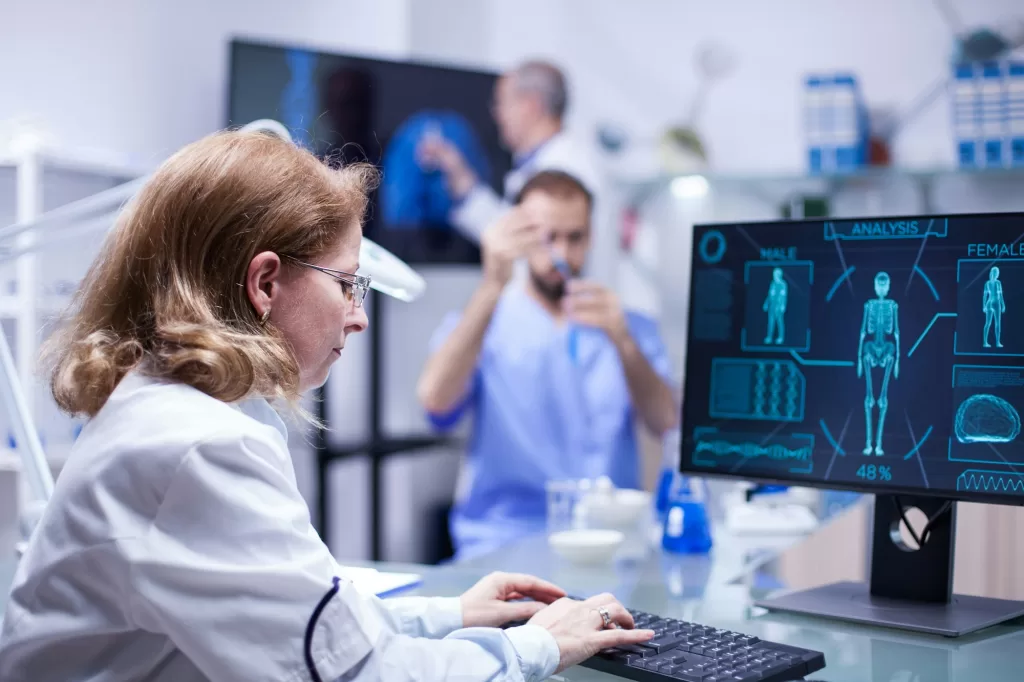
Who Needs Biostatistics?
In today’s diverse biological landscape, each subfield requires unique statistical approaches. However, the necessity of statistics spans across all scientific disciplines, from biomedicine to psychology.
As such, a biostatistician is well-equipped to assist in any scientific study which collects data.
A Curated List of My Work in Biostatistics
Industry R&D
In industry, scientists often juggle the needs of diverse stakeholders, which may not always align. It’s crucial to maintain scientific accuracy while being mindful of cost and time constraints. My pragmatic approach has proven invaluable in these challenging projects.
I’ve collaborated with R&D scientists managing multiple experiments on groundbreaking products, guiding experimental design and analysis. This involves balancing theoretical principles like Design of Experiments theory with practical considerations such as budget and deadlines. Much of this work is confidential due to Non-Disclosure Agreements (NDAs).
Power Analysis
Prior to initiating a study, scientists are often told to conduct a power analysis to ascertain the necessary sample size for meaningful results. While numerous tools and tutorials cater to conventional cases, I’m frequently confronted with projects that don’t fit into a particular textbook case of power analysis. This is where my statistical expertise becomes invaluable, making grant proposals stand out amidst the competition.
- I’ve helped a cognitive psychologist interested in testing an equivalence hypothesis understand that Equivalence Testing was the answer to their question. I then calculated the proper sample size required and helped write their grant proposal for the project.
- I’ve helped a social scientist who intended to collect large amounts of survey data understand how to properly determine the required size of the sample group to be surveyed to make results meaningful.
- A project necessitated a return to the laboratory due to the high cost of required measurements. Tasked with determining an appropriate sample size in the absence of reliable effect-size estimates, I devised a novel approach. By leveraging a small sample containing both the variable of interest and a proxy variable, I estimated their correlation. Utilizing bootstrap distributions, I generated plausible power curves, extracting actionable insights despite the inherent uncertainty surrounding the proxy variable’s correlations.
Randomized Controlled Trials (RCTs)
Randomized Controlled Trials (RCTs) are renowned as the gold standard of scientific inquiry when conducted meticulously. However, their execution is far from routine or simplistic, requiring careful consideration of numerous complexities during study design.
- In a multi-year study on wheat crop yields that I worked on, a fully factorial randomized design was employed, necessitating the resolution of issues stemming from heavy-tailed, strictly positive (non-normal) data. These challenges were addressed through the application of a Gamma Generalized-Linear Model (GLM). Additionally, nutrient concentration variables were modeled as proportions using beta regression. The presence of outliers and influential data points was managed by assessing metrics such as Studentized Difference of Fits (DFFITS), Difference in Betas (DFBETAS), leverage derived from the hat-matrix, and Covariance Ratio. Sensitivity analyses were conducted to comprehensively understand these outliers.
- I aided a team of psychiatrists in determining an appropriate methodology using linear mixed-models to analyze a small trial investigating ketamine treatments for treatment-resistant depression. Given the complexities arising from factors like small sample sizes, longitudinal data with dropout, etc., the team sought guidance on their approach, initially considering Structural Equation Models (SEM).
- I contributed to drafting a protocol for a within-patient randomized controlled trial evaluating a novel dermatological treatment for melanoma, intended for submission to a granting agency. Additionally, I analyzed longitudinal outcomes from a within-patient randomized controlled trial focusing on skin tissue treatments for burn victims, adhering to updated Consolidated Standards of Reporting Trial (CONSORT) guidelines.
- I served as an independent biostatistician on a Data Safety and Monitoring Board (DSMB), tasked with compiling descriptive data summaries and preliminary study results.
Predictive Modeling and Machine Learning
- I’ve developed a classifier model aimed at evaluating the predictive efficacy of select biomarkers concerning kidney transplant rejections. This initiative holds promise for furnishing less invasive diagnostic tools by leveraging biomarker sampling as opposed to conventional biopsy procedures.
- I’ve worked with a cutting-edge dimensionality reduction technique specifically designed to handle complex biological data called Potential of Heat-diffusion for Affinity-based Trajectory Embedding (PHATE) which served as a low-dimensional embedding that could then be clustered using an algorithm such as K-means.
Large Observational Studies in Epidemiology
It is widely known that observational data cannot inherently establish causal relationships from observed associations (correlation =/= causation). Nonetheless, there’s a rapidly evolving scientific revolution underway in Causal Inference, a statistical subfield dedicated to establishing theoretical approaches to estimating cause-and-effect relationships.
- I’ve built and validated a Propensity Score (PS) model, rooted in a comprehensive understanding of counterfactual variables elucidated through Directed Acyclic Graphs (DAG) in collaboration with subject-matter experts. This PS model was integrated into the primary analysis to assess the causal impact of COVID-19 infection on specific neurological biomarkers indicative of brain damage. Given the prevalence of missing data in such large-scale studies, I successfully applied cutting-edge Multiple Imputation (MI) techniques, leveraging substantial expertise in both statistics and computer programming.
- Case-control studies are notoriously difficult designs in epidemiological research. I’ve navigated these delicate intricacies by employing density sampling in a substantial case-control study investigating the evolution of the COVID-19 pandemic within a population of HIV-positive individuals. Utilizing multivariable logistic regression models and proportional-odds regression, I accurately estimated adjusted odds ratios and modeled odds ratios for outcome variables on an ordinal scale, respectively.
Time-Series Models
I’ve successfully employed a complex class of statistical models known as Generalized Linear Autoregressive Moving Average Models (GLARMA) to investigate the temporal evolution of Canadian Media’s compliance with reporting guidelines regarding suicide incidents. Given the discrete nature of the data involved, this study introduced an additional layer of complexity to time-series analysis typically conducted with continuous variables.
- In a large collaboration involving over 40 scientists from diverse fields (biologists, machine learning specialists, bioinformaticians, etc.), I contributed to designing complex estimates for specific questions related to high-dimensional immunological data. Using pharmacodynamics and pharmacokinetics-based Dose-Response curves, along with Generalized Additive Models (GAMs) allowing flexible fits of smooth functions, supported by bootstrap estimates.
- In a project that was threatened by a scathing peer review, I swiftly revamped the statistical methodology to address issues with multiple non-parametric tests. By introducing linear-mixed models and adjusted post-hoc tests, the paper was successfully accepted and published in a prestigious journal.
This selection of examples demonstrates the indispensable role of biostatistics in scientific research across diverse fields. Now, it’s your turn to use the power of biostatistics for your own projects!
Don’t miss out on the opportunity to advance your research with expert biostatistics consultant services. Reach out now and let’s make a difference together!